In a groundbreaking development for North African language technology, Awar AI is proud to announce the release of the first-ever Automatic Speech Recognition (ASR) model for the Tarifit language. This milestone represents a significant step forward in preserving and digitizing indigenous languages in North Africa through artificial intelligence.
Who We Are?
At Awar AI, we stand at the forefront of AI innovation with a clear purpose: bridging the technological divide for underrepresented languages. As a pioneering AI startup, we specialize in developing sophisticated language models that give voice to communities in Middle East and North Africa often overlooked by mainstream technology.
Our team of dedicated AI researchers, linguists, and engineers works tirelessly to create AI solutions that truly understand and represent the nuances of North African and Middle Eastern languages. By focusing on Tarifit, Tamazight, Tachelhit, Moroccan Dialect (Darija), and Gulf Arabic, we’re not just building technology – we’re preserving linguistic heritage for future generations.
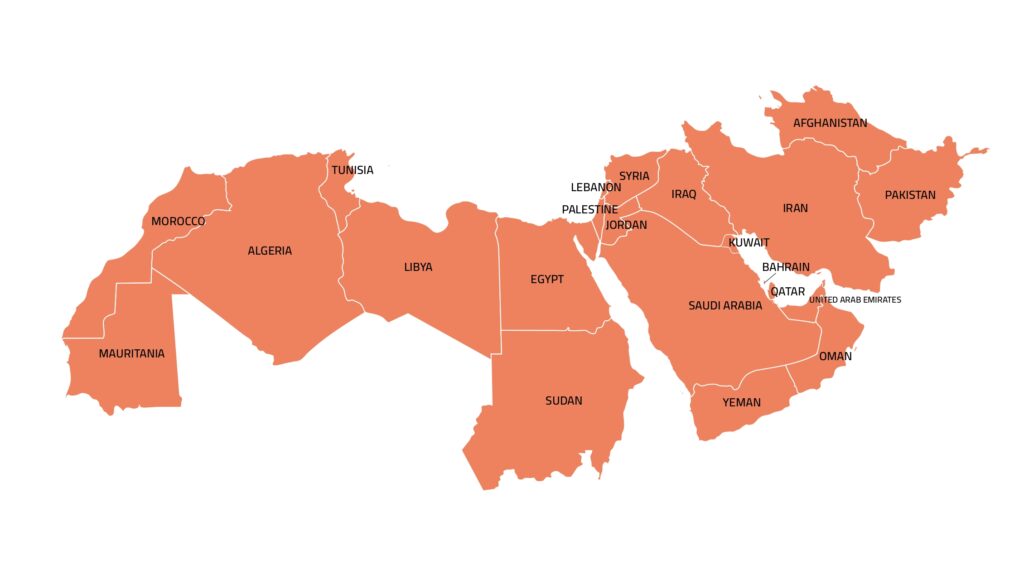
Why Tarifit?
The Tarifit language community represents a significant yet underserved linguistic group in the digital age. With 5 million speakers worldwide, including 3 million in Northern Morocco and major communities across Europe – notably in Belgium (700,000), Netherlands (600,000), France (300,000), and Spain (220,000) – this vibrant community lacks access to modern language technologies that many other languages take for granted.
With over 5 million speakers across Morocco and Europe, Tarifit represents a significant language community that faces critical challenges in today’s digital world:
- No access to modern voice technologies
- Limited digital education resources
- Barriers to cultural preservation
- Lack of automated language services
This digital exclusion threatens both daily communication and language survival, affecting millions of speakers across generations. Our ASR model is a vital step toward ensuring this vibrant community can embrace modern technology while preserving their linguistic identity.
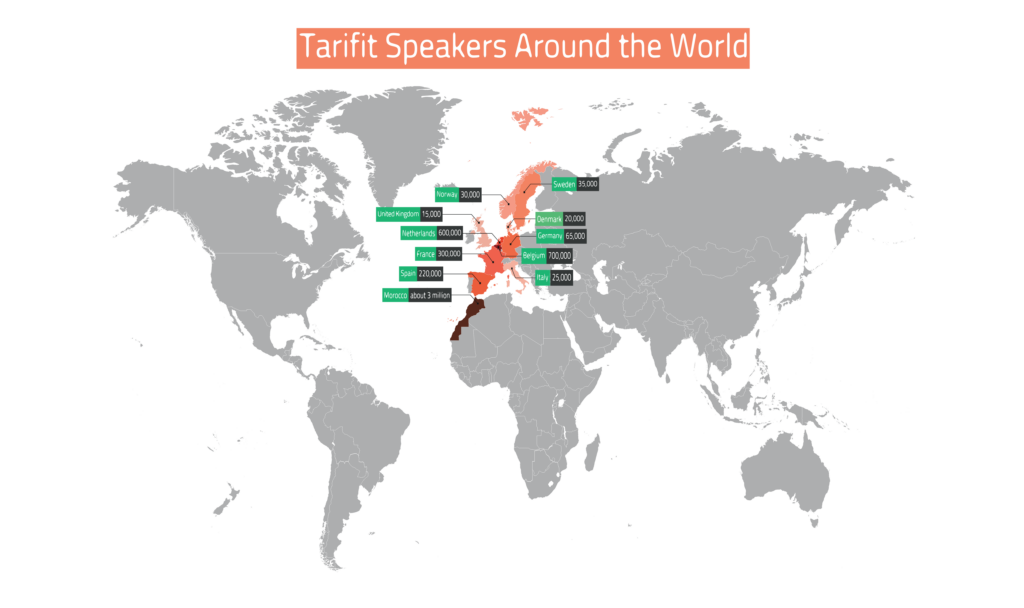
Our ASR
AWAR ASR is the first reliable speech recognition system dedicated to the Tarifit language, transforming spoken Tarifit into accurate written text in real-time. This breakthrough makes digital communication accessible to millions of Tarifit speakers worldwide.
At the core of our technology is a custom neural architecture specifically engineered for Tarifit’s unique linguistic features. Our model has been trained on diverse speech patterns, optimized for real-time processing, and designed to handle regional variations and accents.
From educators creating learning materials to businesses serving Tarifit-speaking customers, our system enables seamless conversion of spoken Tarifit into searchable, shareable text. Built from the ground up with Tarifit in mind, AWAR ASR ensures accurate recognition of distinct phonological patterns and morphological structures, while maintaining minimal latency.
AWAR ASR serves multiple sectors: educators can create accessible learning materials and transcribe lectures, businesses can implement voice-based customer service, media organizations can transcribe local content, and researchers can document oral histories and support linguistic studies. Our system handles real-time processing with minimal latency, supports regional variations and accents, and includes advanced features like noise reduction and speaker separation.
The technology is designed for both real-time applications and batch processing, making it versatile for various use cases while maintaining high accuracy across different environments and speaking styles.
See AWAR ASR in Action
Experience the power of our Tarifit ASR system in real-world scenarios. Watch how it accurately transcribes different speakers, handles various dialects, and processes speech in real-time with remarkable precision.
Evaluating Tarifit ASR Systems
To provide a comprehensive evaluation of our Tarifit ASR systems, we conducted accuracy tests that compared our transcriptions to those of other leading speech recognition providers, namely Meta, OpenAI’s Whisper, and AWS. The testing was conducted on diverse voice samples from native Tarifit speakers from the Rif region.
We used the Character Error Rate (CER) metric, which determines the percentage of characters that differ between the ASR output and the ground truth transcription. The CER is calculated by dividing the total number of errors (substitutions, deletions, and insertions) by the total number of characters in the ground truth transcription.
This benchmark showcases a comparison of the accuracies of various ASR models. The accuracies are simply calculated by subtracting the CER from 100.
Accuracy = 100 — CER
We can see that our system reaches an accuracy of 85%, outperforming other providers in Tarifit speech recognition. This represents a significant improvement in ASR accuracy for the Tarifit language, particularly when handling different regional variations and speaker styles.
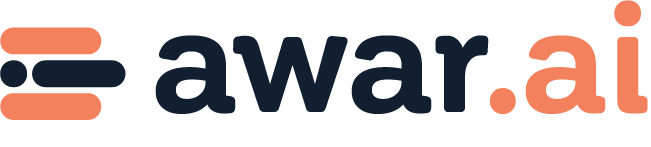
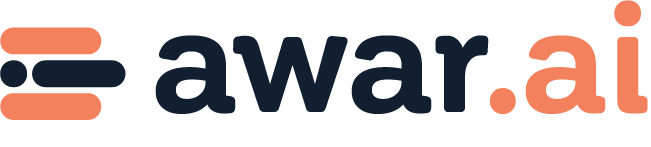
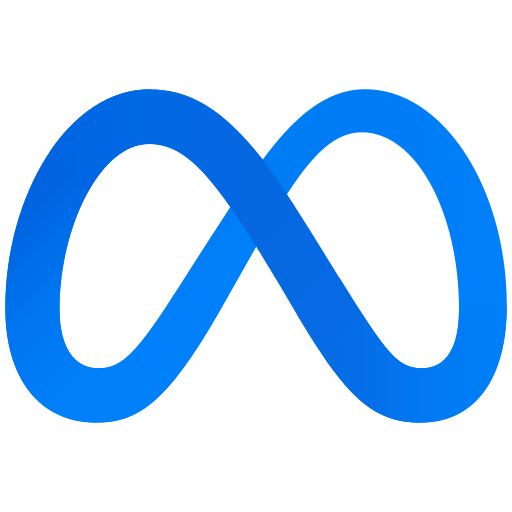
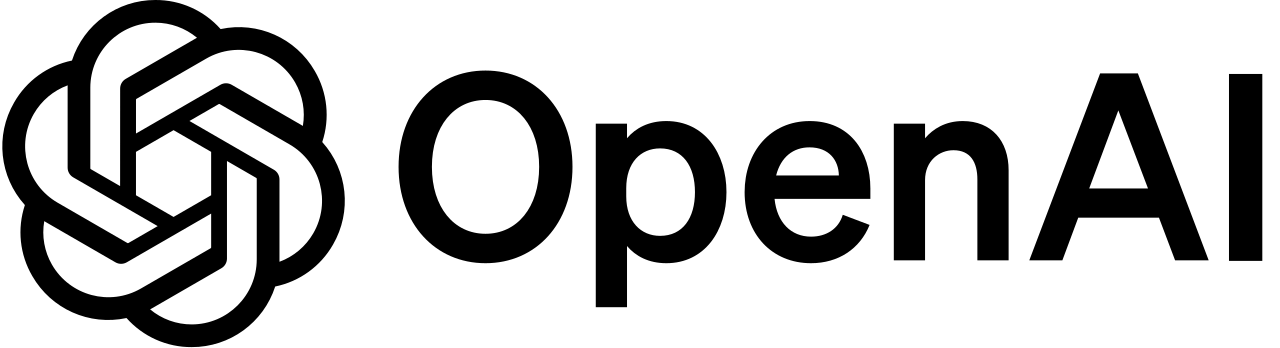
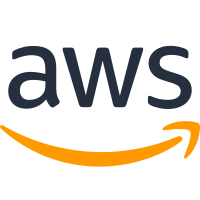
The impact
The arrival of AWAR ASR represents a significant milestone for Tarifit speakers in the Rif region and beyond. By enabling spoken Tarifit conversion into text, our system creates several practical applications:
- Automatic transcription of local radio content
- Voice notes to text conversion
- Digital preservation of oral traditions
- Voice-enabled services for local businesses
- Enhanced accessibility for native speakers
This breakthrough marks a crucial step in bringing Tarifit into the digital age while preserving its cultural heritage. For younger generations, it validates that traditional languages like Tarifit have a place in modern technology, encouraging them to maintain their mother tongue while embracing digital innovation.
What's Next
We will expand our ASR technology to support more regional languages and dialects, including Moroccan Darija, Tachelhit, Tamazight, and Gulf Arabic. This expansion aims to provide the same level of accurate speech recognition across multiple Arabic and Amazigh variants, furthering our mission of making voice technology accessible to more communities in their native languages.